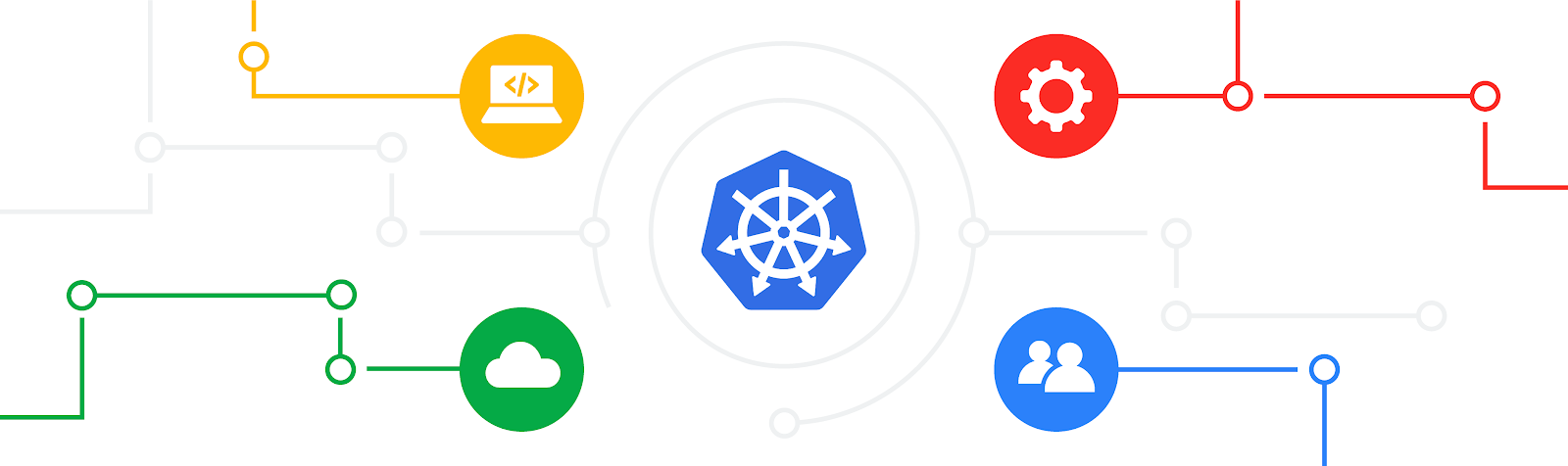
Kubernetes 1.31 is now available in the Google Kubernetes Engine (GKE) Rapid Channel, just one week after the OSS release! For more information about the content of Kubernetes 1.31, read the official Kubernetes 1.31 Release Notes and the specific GKE 1.31 Release Notes.
This release consists of 45 enhancements. Of those enhancements, 11 have graduated to Stable, 22 are entering Beta, and 12 have graduated to Alpha.
Kubernetes 1.31: Key Features
Field Selectors for Custom Resources
Kubernetes 1.31 makes it possible to use field selectors with custom resources. JSONPath expressions may now be added to the spec.versions[].selectableFields field in CustomResourceDefinitions to declare which fields may be used by field selectors. For example, if a custom resource has a spec.environment field, and the field is included in the selectableFields of the CustomResourceDefinition, then it is possible to filter by environment using a field selector like spec.environment=production. The filtering is performed on the server and can be used for both list and watch requests.
SPDY / Websockets migration
Kubernetes exposes an HTTP/REST interface, but a small subset of these HTTP/REST calls are upgraded to streaming connections. For example, both kubectl exec and kubectl port-forward use streaming connections. But the streaming protocol Kubernetes originally used (SPDY) has been deprecated for eight years. Users may notice this if they use a proxy or gateway in front of their cluster. If the proxy or gateway does not support the old, deprecated SPDY streaming protocol, then these streaming kubectl calls will not work. With this release, we have modernized the protocol for the streaming connections from SPDY to WebSockets. Proxies and gateways will now interact better with Kubernetes clusters.
Consistent Reads
Kubernetes 1.31 introduces a significant performance and reliability boost with the beta release of "Consistent Reads from Cache." This feature leverages etcd's progress notifications to allow Kubernetes to intelligently serve consistent reads directly from its watch cache, improving performance particularly for requests using label or field selectors that return only a small subset of a larger resource. For example, when a Kubelet requests a list of pods scheduled on its node, this feature can significantly reduce the overhead associated with filtering the entire list of pods in the cluster. Additionally, serving reads from the cache leads to more predictable request costs, enhancing overall cluster reliability.
Traffic Distribution for Services
The .spec.trafficDistribution field provides another way to influence traffic routing within a Kubernetes Service. While traffic policies focus on strict semantic guarantees, traffic distribution allows you to express preferences (such as routing to topologically closer endpoints). This can help optimize for performance, cost, or reliability.
Multiple Service CIDRs
Services IP ranges are defined during the cluster creation and can not be modified during the cluster lifetime. GKE also allocates the Service IP space from the VPC. When dealing with IP exhaustion problems, cluster admins needed to expand the assigned Service CIDR range. This new beta feature in Kubernetes 1.31 allows users to dynamically add Service CIDR ranges with zero downtime.
Acknowledgements
As always, we want to thank all the Googlers that provide their time, passion, talent and leadership to keep making Kubernetes the best container orchestration platform. From the features mentioned in this blog, we would like to mention especially Googlers Joe Betz, Jordan Liggitt, Sean Sullivan, Tim Hockin, Antonio Ojea, Marek Siarkowicz, Wojciech Tyczynski, Rob Scott, Gaurav Ghildiyal.
By Federico Bongiovanni – Google Kubernetes Engine